Last updated on April 5th, 2023 at 05:20 pm
Data Science is the 21st century’s most difficult job. It has become a buzzword about which almost everyone talks these days.
It’s not easy to become a Data Scientist. To become a Data Scientist, you will need the right resources.
The most common method of learning Data Science is through books.
The real problem lies in choosing the right book. Data Science students are often in a quandary about which book to read.
These books will help you succeed in Data Science if you don’t have the skills to read books.
AI Patasala’s Data Science Training in Hyderabad is the best choice for learning data science online.
Best Data Science Books to Read in 2022
Reading the most recent data science books can give you a headstart. We will discuss the top data science books on the market so that you can add them as part of your 2022 reading list.
1. Data Science from Scratch – Joel Grus
This book will teach you how to implement many of the most important data science algorithms and tools by starting from scratch.
Joel Grus is a mathematician and a programmer. He will help you become comfortable with data science and its mathematics.
2. Head First Statistics: A Brain-Friendly Guide – By Dawn Griffiths
Head First Statistics makes this often-dry subject come alive through interactive, thought-provoking, and engaging material. It is full of stories, puzzles, quizzes and visual aids, and real-world examples.
3. Practical Statistics for Data Scientists – By Peter Bruce and Andrew Bruce
This guide will help you to use various statistical methods in data science. It also teaches you how to avoid misuse of these methods and offers advice about important ones. Although many data science resources include statistical methods, they lack deeper statistical perspectives.
4. Introduction to Probability – By Dimitri P. Bertsekas and John N. Tsitsiklis
This book covers the basics of probability theory (probabilistic models and discrete and continuous random variables and limit theorems) that are often part of a first course in the subject. It also includes the fundamental concepts and methods for statistical inference, Bayesian and classical.
5. Hands-On Machine Learning with Scikit-Learn, Keras, and TensorFlow – By Aurélien Géron
Using concrete examples, minimal theory, and two production-ready Python frameworks–Scikit-Learn and TensorFlow–author Aurelien Geron helps you understand the concepts and tools for building intelligent systems. There are many techniques to learn, from simple linear regression to advanced neural networks. There are exercises in each chapter to apply the knowledge you have gained.
6. Introduction to Machine Learning with Python: A Guide for Data Scientists – By Andreas Muller
This course will teach you how to create a machine-learning application using Python and the sci-kit–learn library. Andreas Mueller, Sarah Guido, and Sarah Guido concentrate on the practical aspects rather than the mathematics behind machine learning algorithms. This book will benefit from your familiarity with the NumPy or matplotlib libraries.
7. Python Machine Learning By Example – By Yuxi (Hayden) Liu
This book is your guide to machine learning. This book provides an introduction to machine learning and the Python language. It also shows how to set up the setup. You will then learn the most important concepts like exploratory data analysis and pre-processing.
8. Pattern recognition and machine learning – By Christopher M. Bishop
This book is the first to introduce the Bayesian perspective in pattern recognition. It presents approximate inference algorithms that allow for fast approximate answers even in cases where precise answers may not be possible. This book uses graphical models for describing probability distributions, which is not available in other books. It assumes no prior knowledge of machine learning or pattern recognition. It is necessary to be familiar with basic linear algebra and multivariate calculus. Some experience in using probabilities would also be beneficial but not required. The book includes an introduction to basic probability theory.
9. Python for data analysis – By Wes McKinney
Python for Data Analysis focuses on the fundamentals of Python data analysis. This book is a modern, practical introduction to scientific computing in Python that is suited for data-intensive applications. This book is about the Python language and the libraries you will need to solve many data analysis problems. This book does not provide an overview of analytical methods that use Python as the implementation language.
10. Build a Career in Data Science – By Emily Robinson and Jacqueline Nolis
Building a Career as a Data Scientist is your guide to landing your first job in data science and becoming a respected senior employee. Follow these simple steps to create a great resume and impress your interviewers. It can be difficult to manage complex stakeholders, keep projects on track and adapt to changing company needs in this dynamic, constantly evolving field. The stories of data scientists who have been there before will help you manage expectations and deal with failures.
11. Naked statistics – By Charles Wheelan
This book clarifies key concepts like inference, correlation and regression analysis. It also reveals how careless or biased parties can manipulate or misrepresent data. And it shows how creative and brilliant researchers are using the valuable data of natural experiments to address thorny issues.
12. Data Science and big data analytics – By EMC Education Services
Data Science and Big Data Analytics are about using the power of data to uncover new insights. This book covers all the methods and tools Data Scientists use. The book focuses on concepts and principles applicable to any industry or technology environment. It also includes examples that you can use with open-source software.
13. R for data science – By Hadley Wickham and Garrett Grolemund
This book will give you a solid understanding of the discipline of discovering natural laws within data structures. You’ll also learn how to use the R programming language for data analysis.
If you measure the same thing twice, you will get two results if you measure accurately enough. This creates uncertainty but also opportunities. Garrett Grolemund is Master Instructor at RStudio. He shows you how data science can help you deal with uncertainty and seize opportunities.
Conclusion:
This concludes this article. I hope these books will add more value to your skillset. Keep growing, keep reading, and keep flourishing.
AI Patasala’s six-month in-person Data Science course in Hyderabad can help you tremendously in your quest to become a successful Data Science professional.
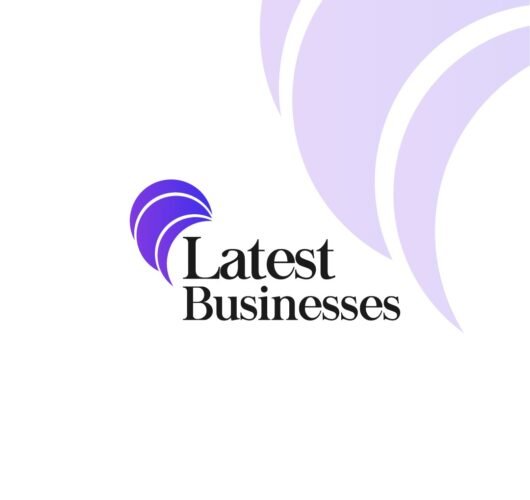
Latest Businesses helps you list your business on every possible business directory in the best possible way to increase your business is seen and recognized. This will increase the reach of your business profile in searches across different search engines.